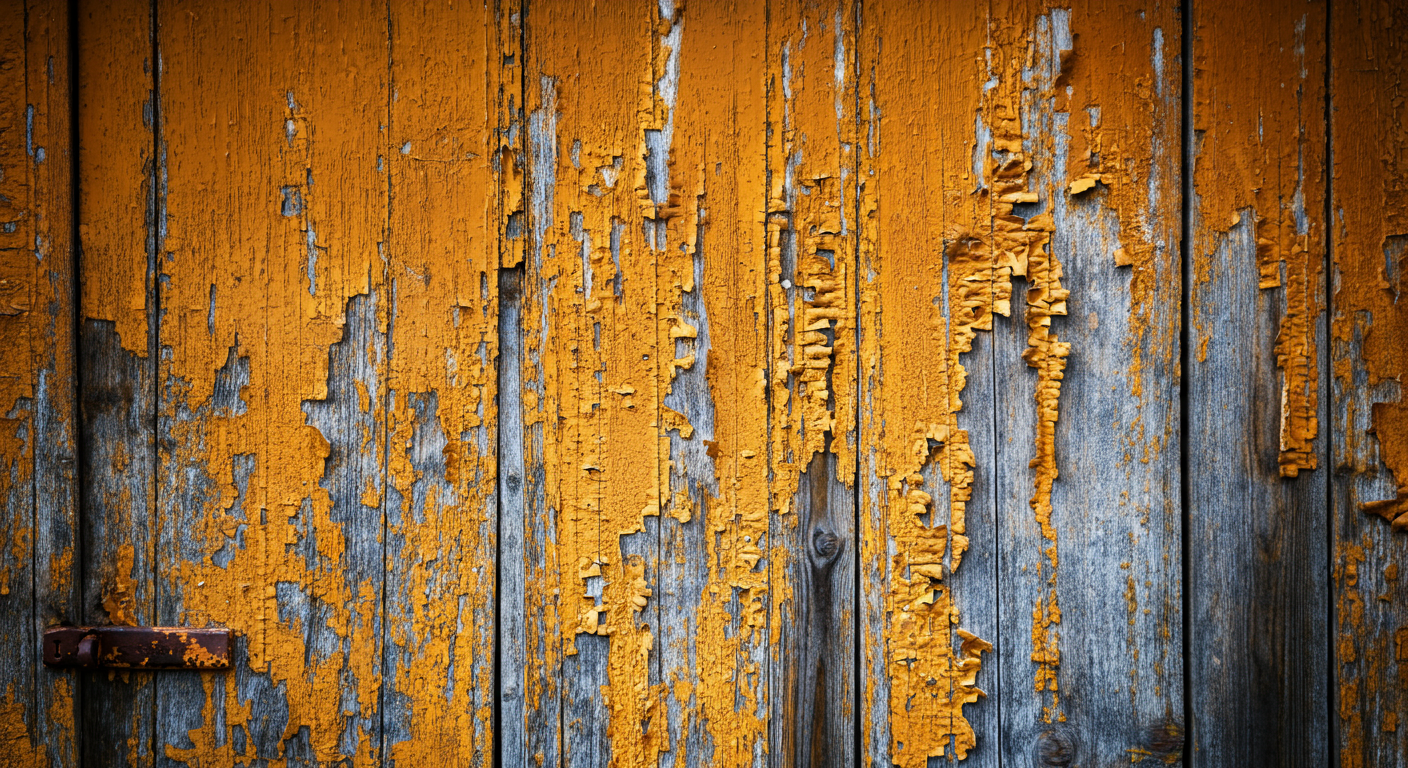
Techniques for Data Validation and Quality Assurance
Built on Evidence, Backed by Method
We apply structured techniques to validate insights across multiple sources, combining AI-assisted outputs with human expertise, peer review, and real-world confirmation. Our process ensures traceable, defendable recommendations by triangulating data from official databases, community input, and policy frameworks.
Our Commitment to Data Integrity
At UPL1FT, we believe that data-powered insights are only as strong as the methods behind them. Whether advising municipal governments or leading national-scale feasibility studies, we deploy rigorous data validation techniques to ensure our findings are accurate, traceable, and transparent.
1. Human-in-the-Loop (HITL) Validation
AI is a powerful tool, but without expert oversight, it can misinterpret nuance or miss contextual cues. We embed human judgment into every stage of AI-assisted analysis. Our subject matter experts continuously review model outputs, correct anomalies, and apply contextual logic, ensuring each recommendation aligns with real-world dynamics and ethical standards (ThoughtSpot, n.d.).
“AI is not a substitute for human expertise. It is a force multiplier. We ensure the final word always rests with people.”
2. Data Traceability and Source Transparency
We implement traceability pipelines that allow every AI-driven insight to be traced back to its original data source. This practice supports transparency, compliance, and stakeholder confidence — especially critical in regulated sectors such as finance, housing, and public policy.
We align traceability with the full data lifecycle: from data ingestion to validation and reporting. All inputs are documented, tagged, and auditable (Encord, 2023).
“Data lineage is more than a technical feature. It is a trust building foundation.”
3. Hybrid Validation Framework
We use a hybrid strategy that combines the speed of AI with the reliability of rule-based verification. Automated data profiling and anomaly detection are followed by traditional cleansing and formatting checks, ensuring that outputs are not just fast, but correct.
Our validation techniques include:
Deterministic and fuzzy matching for deduplication
Format standardization based on pre-set rules
Manual confirmation of anomalies and outliers
This approach reduces false positives and improves confidence in high-stakes decisions (Data Ladder, n.d.).
“AI can suggest. Our team confirms.”
Our approach to data validation is more than technical. It is ethical, accountable, and deeply collaborative. Every model, insight, and dashboard we build is backed by proven validation techniques that balance automation with human expertise.
This is how UPL1FT ensures our clients are not just AI enabled. They are AI ready.
References
Data Ladder. (n.d.). The role of AI in data quality management. https://dataladder.com/the-role-of-ai-in-data-quality-management/
Encord. (2023, August 18). Data visibility and traceability. https://encord.com/blog/data-visibility-traceability/
ThoughtSpot. (n.d.). Human in the loop. https://www.thoughtspot.com/data-trends/artificial-intelligence/human-in-the-loop